Unlocking the Future of Enterprise AI: Insights and Innovations from the Field
Andreessen Horowitz recently released a comprehensive report delving into the dynamics of AI adoption within enterprises, drawing on insights from interviews with numerous enterprise leaders. This blog aims to highlight and discuss the key findings from the report that resonate with our experiences in implementing transformational Generative AI solutions across enterprises.
Implementing and scaling generative AI requires the right technical talent, which currently isn’t in-house for many enterprises.
The implementation and scaling of generative AI in enterprises hinge crucially on having the right technical talent, a resource which many enterprises don’t have internally. The complexity of deploying non-API based models requires a significant degree of technical know-how. This gap in talent is not merely an operational challenge but a strategic bottleneck, limiting the pace at which enterprises can adopt and leverage generative AI solutions. This explains why consultancies like Accenture have seen such impressive GenAI revenue numbers who are able to offer alternatives to in-house development teams.
“Because it’s so difficult to get the right generative AI talent in the enterprise, startups who offer tooling to make it easier to bring genAI development in house will likely see faster adoption.” - A16Z
In response, the market has seen a rising demand for platforms and tools that simplify generative AI integration, enabling companies to bridge the talent gap internally. Something that we have seen at TitanML, our clients are using our platform to make working with open-source models as easy as using API based models, all while retaining the privacy benefits of deploying privately.
““Their platform has completely simplified the once herculean task of LLM deployments. The technology TitanML offers has drastically curtailed the setup time of deployments from a protracted span of a week, to a phenomenal speed of less than an hour” - Hannes Hapke, Machine Learning @ Digits.
Interoperability: Embracing a Multi-Model Future
As enterprises dive deeper into generative AI, the landscape is evolving towards a multi-model future where reliance on a single provider is fading. This shift is driven by the converging performance of various models and the recognition that different AI solutions offer unique advantages for specific applications. The best RAG application for instance, requires 3 models for 3 different providers, one for the embedding, one for the re-ranker, and one for the generation. This diversity in model usage introduces significant complexity in managing interoperability among them, necessitating varied infrastructure setups to accommodate each model's unique requirements.

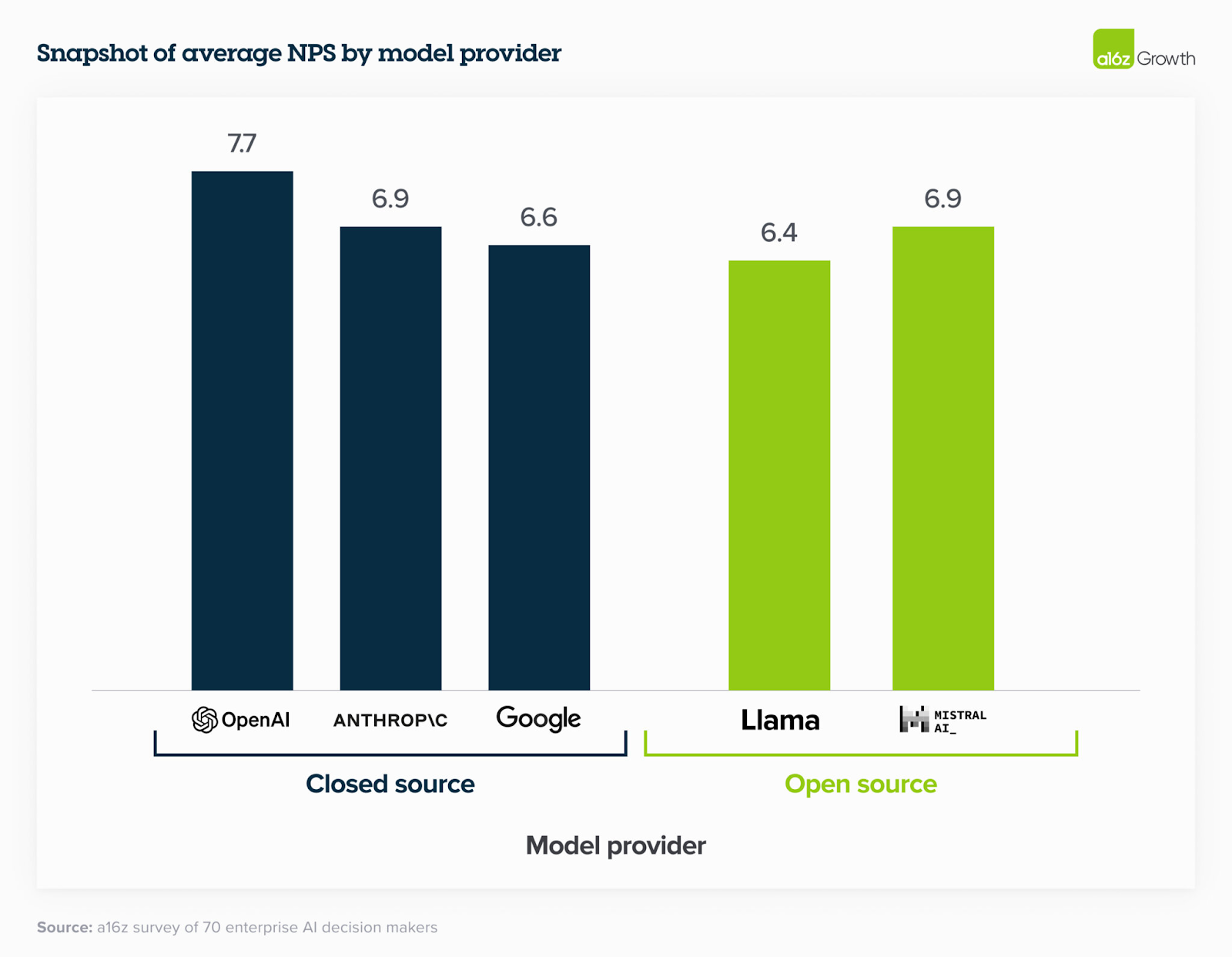
Acknowledging this challenge, at TitanML we have worked to streamline the multi-model deployment process, making it easier for companies to stay at the forefront of AI advancements without constant infrastructure re-engineering. By simplifying the adoption of the latest models to changing a single line of code, at TitanML we enable enterprises to seamlessly deploy applications and keep them up-to-date with the best available technology. The introduction of our OpenAI compatible API further enhances this capability, allowing users to effortlessly integrate both open-source and proprietary models, thereby fostering a truly flexible and adaptable AI ecosystem within their operations.
Open-source Models are the Long Term Strategy for Privacy and Control
The trajectory of enterprise AI adoption is markedly steering towards open-source technologies, with an overwhelming 82% of enterprises projecting an increased reliance on these platforms in the long term. Initially, many organisations leveraged platforms like OpenAI for proof-of-concept projects and to garner early value from AI applications. However, as these enterprises look ahead, there's a growing preference for open-source solutions, driven by a need for more scalable, controllable, and secure AI frameworks.
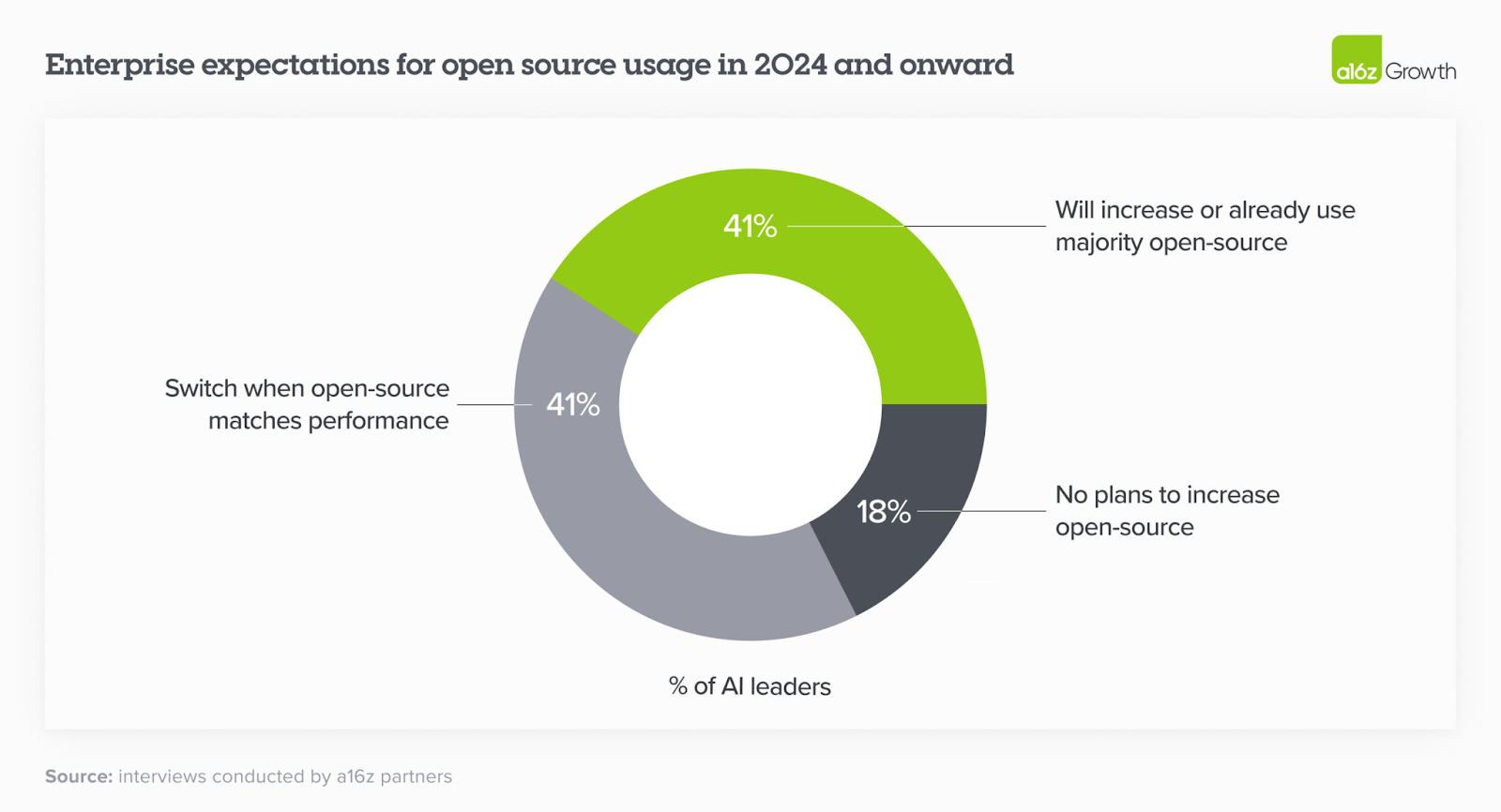
“Because we know that the TitanML Takeoff infrastructure is secure and robust, our internal teams can focus on putting the finishing touches on impactful AI applications” - Stephen Drew, Chief AI Officer @ Ruffalo Noel Levitz
Bespoke, Tailored AI is Delivering the Biggest Competitive Advantage
A16Z identified that enterprises are increasingly opting to build their own AI applications rather than purchasing off-the-shelf applications. This focus is intuitive considering the biggest advantage of AI adoption comes from leveraging proprietary data and applying AI to proprietary workflows - something that is not possible when buying off-the-shelf applications. This is why enterprises have identified building internal solutions as being their best route to competitive advantage. The emphasis on internal use cases, deemed safer and more directly beneficial for competitive advantage, underscores this trend.
“Because these concerns still loom large for most enterprises, startups who build tooling that can help control for these issues could see significant adoption.” - A16Z
Interestingly, while enterprises are leaning towards creating bespoke AI solutions, there's a concurrent investment in the infrastructure that facilitates such development. This approach allows enterprises to tailor AI functionalities to their specific needs while avoiding the extensive time and resource investment that building from scratch would entail. This is just one of the reasons why our clients use TitanML infrastructure and technology to gain a competitive advantage.
“By acquiring this pre-build solution you save valuable time and resources that would otherwise be spent on developing and maintaining in-house infrastructure” - Joe Hoeller, Head of Data Science @ US Healthcare
Knowledge Management: A High Value AI 101 Project for Enterprises
A16Z identified knowledge management as one of the key areas of experimentation for enterprises, with 60% of enterprises experimenting with this use case.
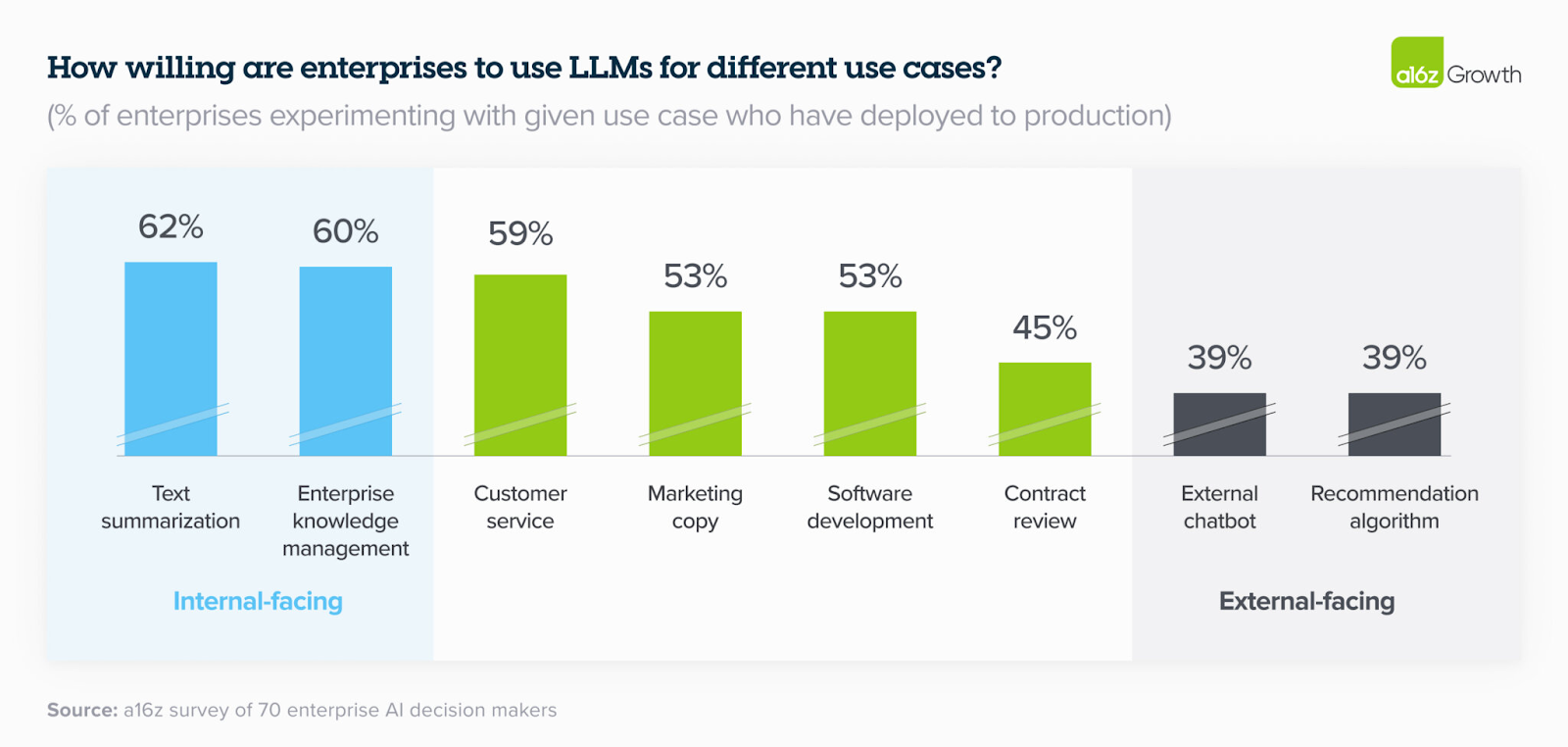
This is a use case that we have seen great success with at TitanML. A great example of this application delivering value is the partnership between Ruffalo Noel Levitz (RNL) and TitanML.
“RNL worked with TitanML to create a privately hosted AI powered knowledge retriever to improve the efficiency of internal operations teams. The Titan Takeoff RAG engine allowed us to get to value within weeks, allowing to to start from a point of already robust and scalable infrastructure” - Stephen Drew, Chief AI Officer @ Ruffalo Noel Levitz.
Want to explore how your Enterprise can adopt Generative AI rapidly, securely, and salably then reach out to hello@titanml.co
Deploying Enterprise-Grade AI in Your Environment?
Unlock unparalleled performance, security, and customization with the TitanML Enterprise Stack