Exactly 363 days ago, I made a few predictions about what 2024 might hold for us. Now, it’s time to evaluate how those predictions fared and what lessons we can draw for 2025.
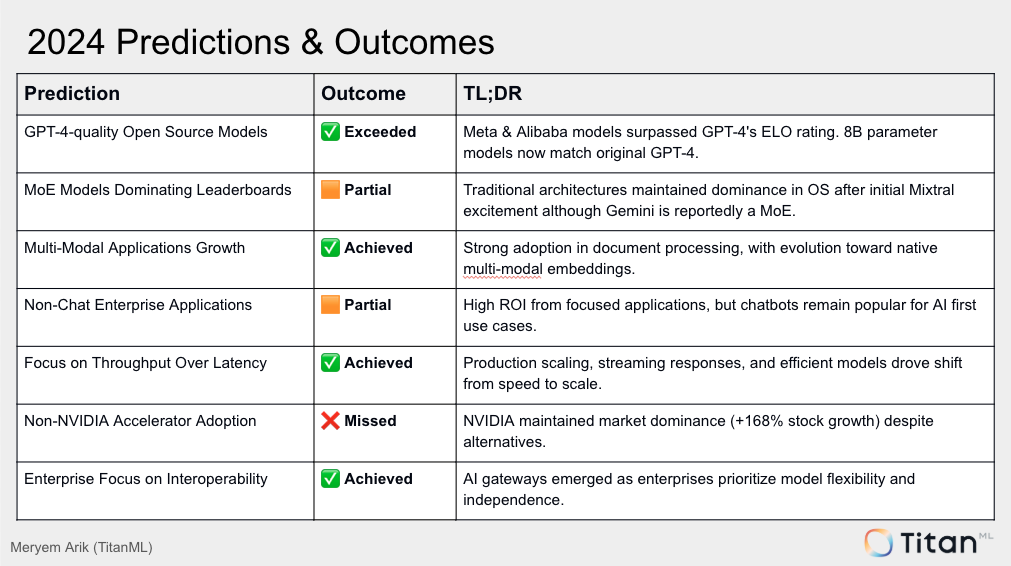
Prediction 1: GPT-4-quality, but with an open source model ✅
This prediction not only came true but was surpassed in unexpected ways. The rise of open-source models in 2024 has been remarkable, driven largely by organizations like Meta (with their LLaMA series) and Alibaba (with the Qwen models).
For context, the original GPT-4 model (GPT-4-0314) achieved an ELO of 1183, whereas Meta’s LLaMA-3.1-405B-Instruct-fp8 and Alibaba’s Qwen-Max-0919 have reached ELOs of 1267 and 1263, respectively.
A standout achievement this year was the development of 8B-parameter open-source models that rival the original GPT-4 in quality. This breakthrough, particularly evident in the LLaMA 3 series, underscores the rapid advancements in open-source AI.
Looking ahead to 2025, we anticipate further progress, with open-source models likely fulfilling 90% or more of enterprise production use cases. Meta’s aggressive investment in this space and the plateauing advancements in proprietary AGI labs make this trajectory highly plausible.
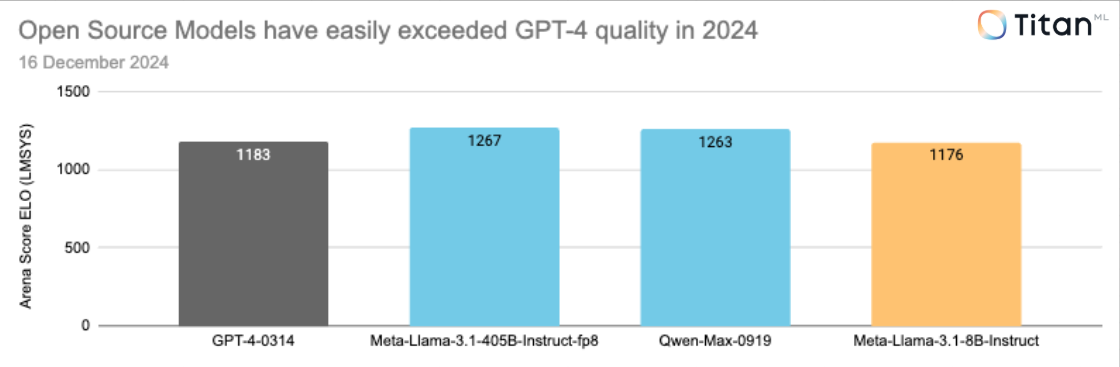
Prediction 2: Mixture of expert (MoE) models dominating open source leaderboards 🟧
This prediction fell short. After the brief surge of interest in Mixtral from Mistral in late 2023, the MoE approach has largely faded from the spotlight. Instead, standard architectures continue to dominate open-source leaderboards, delivering consistent and predictable performance. However, in the closed source space - it is reported that many of the leading foundation models are MoE such as Gemini (3).
Prediction 3: Multi-Modal applications increasing in popularity ✅
This prediction held true. Multi-modal models have increasingly been adopted for complex document processing tasks, particularly within enterprise contexts. These models now handle visual materials—such as strangely formatted tables and PowerPoint slides—with impressive accuracy.
A notable trend is the use of multi-modal models to first “describe” visual inputs (charts, graphs, or images) before running inference on the resulting text description. However, this translation step can introduce some loss of information.
For 2025, we expect wider adoption of natively multi-modal embeddings, allowing enterprises to bypass this lossy step entirely and achieve even greater efficiency.
Prediction 4: Enterprise applications will be dominated by non-chat applications 🟧
Mixed results here. While chatbots dominated 2023, their ROI for enterprise use cases often proved minimal. In contrast, focused applications—such as document processing and summarization—delivered the highest returns for organizations already advanced in AI adoption.
However, many enterprises still view chat-based applications as an accessible entry point to demonstrate AI adoption. This trend may persist in 2025, although as organizations move in the maturity curve they likely prioritize higher-impact, specialized tools.
Prediction 5: Decreased focus on latency and increased focus on throughput and scalability ✅
This prediction has proven accurate for several reasons:
- Production Scaling: As AI applications reach production-scale deployment, system performance priorities have shifted to ensure scalability.
- Streaming Responses: Streaming is now a standard UI paradigm, significantly reducing latency demands. The acceptable latency threshold is now simply "faster than reading speed."
- Smaller Models: Today’s “good enough” models are much smaller (e.g., 8B parameters or fewer), reducing latency bottlenecks and improving efficiency.
For 2025, we expect continued emphasis on scalability and throughput as enterprises further optimize their AI-serving infrastructure.
Prediction 6: More inference is done on non-NVIDIA accelerators like AMD, Inferentia, and Intel ❌
This prediction did not materialize. NVIDIA’s dominance persisted, reflected in its stock performance (+168%) compared to Intel (-55%) and AMD (-9%) in 2024.
Nonetheless, there is growing interest among enterprises in designing AI stacks with hardware interoperability. Many organizations aim to future-proof their infrastructure, ensuring flexibility to switch between NVIDIA and alternative hardware in the long term.
Prediction 7: Enterprises build applications with interoperability and portability in mind ✅
This prediction was spot-on. In 2024, the rise of AI gateways allowed enterprises to treat large language models (LLMs) as interchangeable commodities. With rapid advancements in LLMs, businesses have recognized the value of building systems that can seamlessly switch between models to maintain performance and independence.
We expect this trend to strengthen in 2025 as enterprises continue to adopt adaptable AI frameworks to stay ahead in a competitive landscape.
Stay tuned as we share our predictions for 2025 and how your enterprise can stay ahead of the curve!
(1) https://lmarena.ai/ as of 16th December 2024
Deploying Enterprise-Grade AI in Your Environment?
Unlock unparalleled performance, security, and customization with the TitanML Enterprise Stack